Secret LLM chickens II: Tuning the chicken
· 17 min read
When working with an LLM, sometimes it doesn't generate responses in the way you want. Maybe it's being too creative and weird when tasked with serious prompts ("Write me a cover letter for a programming job" "I am a coding wizard and I always min-max my character!"), or it's being too serious when you want to do some creative writing ("Write me a story" "You are tired and you lie down and go to sleep. The end."). This can be tweaked by making certain adjustments to the sampling mechanism—aka "the chicken."
This blog post continues from my previous article, The secret chickens that run LLMs, and you should read that first to understand what a "stochastic chicken" is.
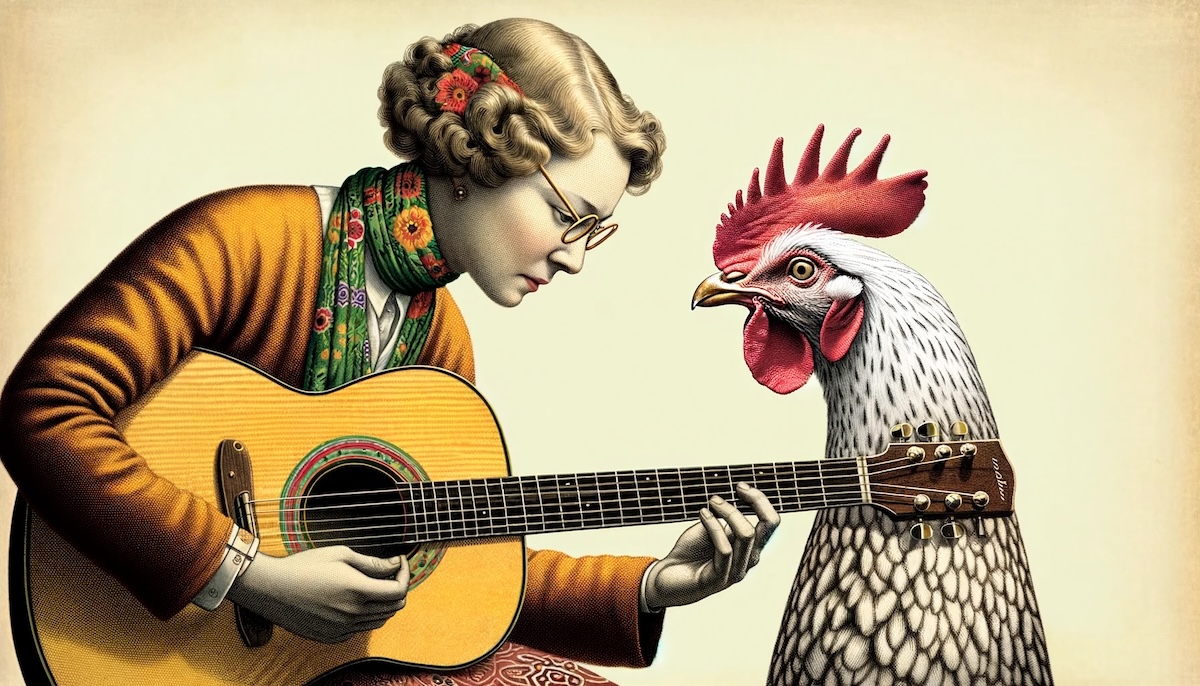
Some key points I'll address here are:
- The "chicken" can be tuned, using inference hyperparameters like temperature, top-k, and top-p. These serve as dials to fine-tune the randomness introduced by the stochastic process, balancing creativity and coherence in the text they generate.
- Adjusting the temperature parameter can make the model's outputs more predictable and less random at lower values, or more diverse and less deterministic at higher values.
- Modifying the top-k and top-p parameters fine-tunes the sampling process by limiting the set of possible next words.
- Top-k restricts the model to choose from the most likely next words, while top-p uses a probability threshold to create a dynamic set of options. These tweaks help balance creativity with coherence, allowing the LLM to better meet specific needs or experimental conditions.
- Even when using top-k, the astronomical number of possible text sequences challenges the idea of detecting originality and plagiarism. It's nearly impossible to prove the source of any specific piece of text generated by these models, although LLM-generated text can be recognizable due to the language and style used.